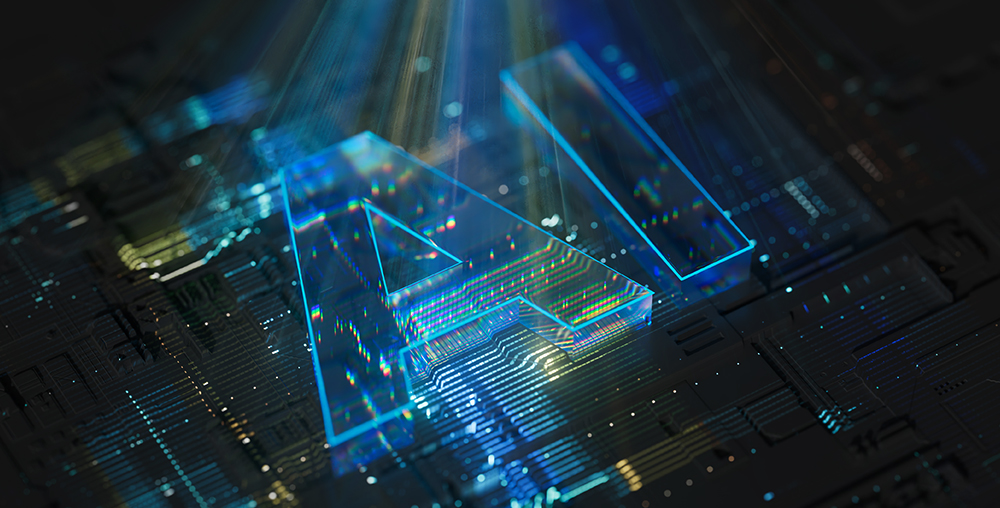
In the age of big data and artificial intelligence, the insurance industry finds itself at a crossroads between innovation and ethical responsibility. During a webinar with Wisconsin School of Business Risk and Insurance Department Chair Daniel Bauer, we explored the complex issue of algorithm bias and fairness, shedding light on its implications for insurers and consumers alike.
The Impact of Past Decisions
Past claims data and external consumer data play a pivotal role in shaping insurance rates and coverage decisions. However, these data sources may harbor inherent biases that pose a significant challenge. As algorithms analyze historical data to inform future decisions, they risk perpetuating and amplifying existing biases, ultimately undermining fairness principles.
For example, if past insurance decisions – e.g. whether to decline a certain policy application – were influenced by factors such as race, gender, or socioeconomic status, algorithms trained on this data may inadvertently incorporate and reinforce these biases, leading to unfair outcomes for certain demographic groups.
Addressing algorithmic bias requires a multifaceted approach that begins with an acknowledgment of the problem. Insurers must first recognize the potential for bias within their data and algorithms, and they must take proactive steps to identify and mitigate these biases. This may involve conducting comprehensive audits of algorithmic decision-making processes, scrutinizing data sources for potential biases, and implementing safeguards to prevent biased outcomes. However, as Professor Bauer’s webinar explained, this is easier said than done.
Legislative Response: A Possible Step Towards Accountability
Recognizing the need to address potential problems with AI solutions such as algorithmic bias, legislative bodies have begun to act. One notable example is Colorado Senate Bill 21 169, which aims to protect consumers from unfair discrimination in insurance practices. The proposal asserts that holding insurers accountable for testing their algorithms and predictive models presents a critical step towards ensuring fairness and transparency in the insurance industry.
The passage of legislation such as Senate Bill 21 169 reflects a growing awareness of the potential harms posed by algorithmic bias in insurance. However, insurers also acknowledge that their practices have important consequences for consumers. So, ascertaining a fair and well-functioning insurance marketplace is a concern beyond simply checking regulatory checkmarks.
Balancing Procedural and Distributive Fairness
Unfortunately, it is not trivial or obvious how to design practices and regulations. When it comes to algorithmic fairness, there is no one-size-fits-all solution. As insurers strive to optimize accuracy while minimizing bias, they must navigate the delicate balance between procedural and distributive fairness.
Procedural fairness revolves around the idea of equal treatment. It emphasizes the importance of fair and transparent decision-making processes, ensuring that all individuals are subject to the same rules and procedures.
In the context of insurance, procedural fairness means insurers should adhere to consistent and unbiased practices when assessing risks and determining premiums. For instance, insurers should apply the same criteria and procedures to all policyholders, regardless of their demographic characteristics or other personal attributes.
In contrast, distributive justice focuses on the fairness of outcomes and distributions within a certain population. It seeks to ensure resources, opportunities, and benefits are distributed equitably among all members of the population.
In the context of insurance, principles of distributive fairness imply that an individual should not have their application declined or be charged higher rates based on their race, gender, or socioeconomic status. For instance, this may be taken to mean that the rate of low risks that are erroneously classified as high risks by a predictive algorithm should be equal across different racial groups.
Principles of distributive justice require insurers to consider the broader societal implications of their underwriting and pricing decisions.
The key difficulty in navigating procedural and distributive fairness is that they are in opposition. By mathematical necessity, in most cases, it is not possible to have an algorithm that satisfies both procedural and distributive fairness. It is necessary to take a stance on the tradeoff between the two principles.
Addressing algorithmic bias and fairness in insurance is a complex challenge without a simple solution. “Depending on what insurance line you’re thinking about, fairness might be more or less important. All of this is going to require humans to make judgments,” says Daniel Bauer.
Navigating Ethical Gray Areas
A consequence is that in the quest for eradicating unfair bias and ensuring fairness, human judgment remains paramount. While algorithms can help us accomplish miraculous tasks, as the recent rise of large language models illustrates, they cannot replace the nuanced decision-making capabilities of human beings. As stakeholders grapple with the complexities of algorithm bias and fairness, it is essential to engage in open and honest dialogue, recognizing that there are no easy answers or quick fixes.
Insurance professionals must approach the use of artificial intelligence and machine learning with a nuanced understanding of these complexities. It’s not enough to simply build the most accurate predictive model – you must also consider the ethical implications.